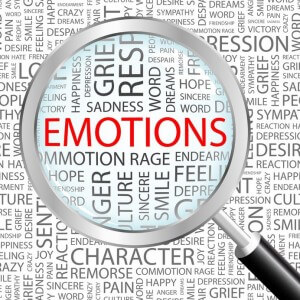
Sentiment
Sentiment analysis has been around for a while, so perhaps it’s about time we looked at the sentiment towards sentiment analysis? I mean is this just a gimmick or is it really ‘of value’.
Negatives
1. The McDonalds effect
If your product or service is ‘bog-standard’ you are likely to be sitting on a mountain of chat-trash. I call this the McDonalds effect as most chatter on McDonalds comes under this guise e.g., ‘hey bob, I’ll meet you round by McDonalds’.
Management implications: quantity of talk does not equate to quality of useable output.
2. The So What? Effect
If today you notice a spike in negative sentiment because people disliked XYZ advert on the TV, that doesn’t mean anything. It doesn’t mean you will lose sales. It just means a bunch of people didn’t like a TV ad. Unfortunately textual analysis although able to identify ‘potential’ root causes will still not be able to quantify the effect on value i.e., tell you if you need to be worried or not.
Let me give you another example.
If you have a piece of text from a hotel stay and it says some negative things about the bed and some slightly negative things about the internet connection that does not mean that you can say anything about the overall stay (i.e., as being positive or negative). For instance, these two items may be negative but be so minor as to not impact my overall satisfaction. Likewise the slight negative of the internet connection maybe much more of a serious thing than the more intensive negative feeling felt towards the bed. You cannot net to the two offer based on the individual emotion word.
Consider a grocery store that puts on a display of cooking, ‘wow! I feel emotional’, in the same store ‘I like’ (in a light flicker of emotion kind of way) the price of those jars of honey, so I buy them. The cooking display does not relate to value (although may act indirectly) to the customer or the firm; the slight feeling of liking the price of honey does. It’s not the emotion that counts but what it means,
Management implications: do not take sentiment at face value. If you do you could be running around wasting time and money fixing things that don’t need fixing, worrying about things, that don’t need worrying about.
3. Issues of representativeness or let’s just focus on the bitchin few
Just because it’s from a big pool of people online doesn’t mean that it adequately represents your customer base. What it represents is those people who are prepared to go online and chat about you, mostly in a negative ‘bitchin’ kind of way. The rules (however flexible) of statistics in terms of representativeness to your customer base do not magically disappear. So my company feels bland today so what, am I bothered it’s just those bitchin customers talking again,
Management implications: do you want to base your corporate decisions on the few people who post vaguely spiteful comments about you. That seems nuts. If most people don’t care about the issue forget it. You could be just basing your business decisions on a few ‘outlier’ crazies that are usually deleted in a quantitative dataset as ‘unrepresentative’.
4. People don’t talk about the things they don’t know about, even if those things are important to how they behave
One of the big disadvantages of textual analysis is that you are limited to what people say! To give an example, when we conducted our original work on emotion we found that a key word emotion shoppers were concerned about was whether they felt ‘relaxed’ or not. That sounded reasonable in the hustle and bustle of a shopping mall. But when we analysed the data statistically we found that levels of relaxation had no effect on business value. Why? Because while it was a straightforward question for customers to answer – how would you like to feel – it was much more difficult for customers to answer – and what emotions and experiences will lead you to spend more money with company X or rate their higher on customer satisfaction and likelihood to recommend.
Management implications: sometimes you just need numbers if you want to predict effects!
5. The problem with machines
I am always deeply suspicious of a machine defining the context of an emotion word. Context is best adjudged by human interpretation.
Management implications: humans are frequently better at understanding context.
6. The medium is the message but not necessarily the truth about how I feel
In my English way I might say, ‘I was a bit disappointed with your service today’ and score slightly negative on sentiment, but if you’d asked me to quantify my disappointment I would have given it a 1 out of 10; which is a truer reflection of long term feelings, the written tweet or facebook comment or the score on a scale.
Likewise, just because I don’t use a strong emotive term, does not mean that I don’t have a strong emotive reaction to your brand. I love Apple, that doesn’t mean to say on every tweet I am going to say I love Apple. I might say, ‘hey bob; meet you by the Apple store’ (sentiment 0%). In the same way, I may be angry with you right now in this tweet, but actually I still love you. It’s only a small matter anyway.
Management implications: text can be a mask to the truth
7. The creation of a neurotic culture
Some guy now gets a goldmine of charts to critique and cajole staff with. Look at this one person used a bad word; our sentiment is 2% below what it was last week. Well perhaps your products boring and the only people who bother to say anything are more likely to use ‘harsher’ language anyway.
Management implications: don’t let the data wonk ruin your day.
Positives
1. A pool of ideas
Although not directly related to sentiment, as an output it is neat at garnering a range of redesign idea from a large set of respondents. Usually it is a challenge to get a mass consumer response to how an experience can be redesigned; this is an excellent ‘crowdsourced’ way to achieve this.
Management implications: use it! There may be some great ideas hidden there. But don’t wholly depend on it; I am sure you have some great ideas of your own.
2. Immediately responsive sentiment monitor
No more long surveys emailed to non-responsive customers, that takes 4 months to analyse by which time the data has gone out of date and the sentiment has changed. This gets the sentiment straight into the company and often in real-time. This means that it is relevant to the immediacy of the situation. In fact, because sentiment can change rapidly it acts as an immediate flag for any potential changes.
Management implications: as a flagging tool I have no issue; saves on the survey. But don’t use it as the only tool.
3. The talkers are the influencers on customer sentiment
Who is to say that the talkers are not the true influencers on consumer sentiment? It may well be that the data is non-representative, but it is representative of those customers most likely to get their voice heard and their feelings felt.
Management implications: difficult one, I would not depend on this wholly but additionally. Look for the strongest sentiments, the real spikes of concern, and more rarely love.
4. Enables a two-way flow on pilots
The great thing about this type of research is that it opens the door to a two-way conversation between customer and researcher. Now we can pilot an idea, get some sentiment back on the proposal and further ideas for change from our pool of respondents.
Management implications: yes, like point 1 on the positives, should be used But not wholly depended upon, I mean you might have some ideas of your own.
5. Breaches all that statistical rhubarb
It’s just damn easier to understand. I can see how we are performing on sentiment, and drill into some of the comments people are saying, and I get a nice dashboard. And a nice dashboard is an important thing. I use it; it makes me feel in control.
Management implications: more attractive than you might think. Culturally puts the frighteners on static reporting, and for my money that’s not such a bad thing.
Overall Sentiment Score -2
Well a little negative as there are more negative comments than positive – and as we have no way of knowing how this has been weighted we’ll have to go with that.
The glaring gap in all of this is the issue of the type of data being worked. I mean, the points raised about the data potentially not representing (a) what the respondent feels and (b) the consumer population of interest are pretty serious. Likewise, the inability to answer the So What? Question. But then again, capturing ideas around sentiment is a major strength as is the immediacy of response.
So some gobbledygook, some bright spots and a nice looking dashboard!
Management Implications
Sometimes you can’t do without numbers.
Add in some questions that capture the quantitative basis for sentiment. Use Net Emotional Value as an easy way to balance positive and negative emotions and relate these back to quantified touchpoint drivers of NEV sentiment.
Steven Walden is VP Consulting and Thought-Leadership for Beyond Philosophy. Steven has 17 years Strategy Consultancy experience directing and designing strategies for major B2C & B2B firms. At Beyond Philosophy, the Global Customer Experience Consultancy, he is a Thought Leader and Innovator, directing engagements to assist leading firms to transform through Customer Experience. A world-leader in emotional experience his skills lie in innovation, thought-leadership, strategy consultancy and Qual/ Quant research. He is a regular speaker at conferences, blog writer, CE Trainer and international author. |